“Correlation doesn’t imply causation.”
That’s a phrase most marketers have had to learn the hard way.
In a complex digital ecosystem, a prospect is exposed to multiple channels before a conversion takes place. It makes perfect sense for app marketers to question: “Would the new users have installed our app even without the ad exposure?”
Incrementality testing or uplift measurement helps marketers assess the real impact of their ad campaigns and channels. In this blog, we look at the need for incrementality testing and how to get started.
Why look beyond the current attribution models?
At the dawn of digital, channels were few, the user journey was straightforward, and measurement was easy. Last-click attribution model made sense for marketers. While the digital space evolved rapidly, attribution models simply did not keep up. The digital space is highly interconnected. In reality, it takes a range of touchpoints, channels, and formats to ultimately influence the desired action from the user.
How do attribution models take into account the full picture? They don’t!
They simply fall short of answering a critical question for marketers: how are campaigns adding value beyond what last click and last view can measure, thereby shedding light on under- or over-attribution of performance?
That’s why marketers must strive for an attribution system that captures the intricacies of brand-user interaction and intelligently paints the full picture. Incrementality testing is one methodology that can help marketers justify their advertising efforts.
What is incrementality testing?
It is a data-driven methodology that measures the direct incremental result of your campaigns by using the principle of Randomized Controlled Trials (RCT).
It identifies the differences in behavior between two audience groups – one that has been exposed to the ads (test group) and one that has not been (control group).
Measuring this difference in conversion rates can help you understand the true impact of your ad campaigns.
Why consider incrementality testing?
Without incrementality testing, your advertising decisions will be based on:
1) Incorrect correlations and assumption dependency
2) Click-focus and not intent–focus
3) Ignorance to immeasurable contributors
Incrementality testing uncovers what campaign or channel investments are actually paying off. It essentially helps you eliminate wastage and shift focus towards the real heroes of your advertising mix.
While it is not a substitute for the pragmatic day-to-day attribution models, it certainly is an additional layer of reassurance for marketers to justify their advertising efforts. Ultimately, it allows you to advertise with clarity and confidence.
Introduction to the types of methods
1) Intent-to-treat method
In this method, a part of the target audience is split into two groups; test and control, with the former receiving brand ads while the latter is withheld from the same. The premise is simple: the conversion/revenue generated from the test group is compared to the one generated from the control group. This allows for a straightforward measurement of ‘uplift’ from your ad campaigns.
Winning factors
🔹 Easy to implement and widely used.
🔹 No additional cost to the advertiser.
Keep in mind: In a programmatic environment, this method doesn’t guarantee that all the test group users are actually exposed to ads since it depends on factors like the win rate and supply availability. However, a sufficiently high exposure rate helps suppress the noise from counting in the results of unexposed users.
2) Placebo Ads/ PSA Ads:
In this method, the test group is shown the brand ad, while the control group is shown a PSA ad or a social service ad. By actually serving ads to both the test and control group, you get clear visibility into the users who are actually exposed to the ads. The uplift is then measured between the test group users who saw the ad vs the control group users who saw the ad.
Winning factors
🔹 Minimal noise and more reliability.
🔹 Easy to implement.
Keep in mind: This method includes additional media costs for running the PSA ad to the control group, and the user targeting has to be equivalent in both groups to avoid bias.
3) Ghost Ads
While this method is considered to be a good combination of Intent-to-Treat and Placebo Ads, it is still in its nascence in the Adtech world and lacks trackable transparency. Here, the DSP platform splits the target group into test and control groups only once the bidding takes place.
In other words, ghost bids are used to mark and exclude control group users and actual winning bids deliver actual brand ads to users in the test group. This means that while the control group does not see any ad, the advertiser has access to the log-level data for when the experimental ad could have been served.
Winning factors
🔹 No media cost for the control group.
🔹 Little noise since the comparison is in between the users who got exposed vs the users who would have been exposed. The remaining noise would be users where the impression could theoretically not be served.
Keep in mind: While this method is great in theory, it is hard to really verify the ghost bid wins since no platform (except self-regulated networks) has access to the entire auction process.
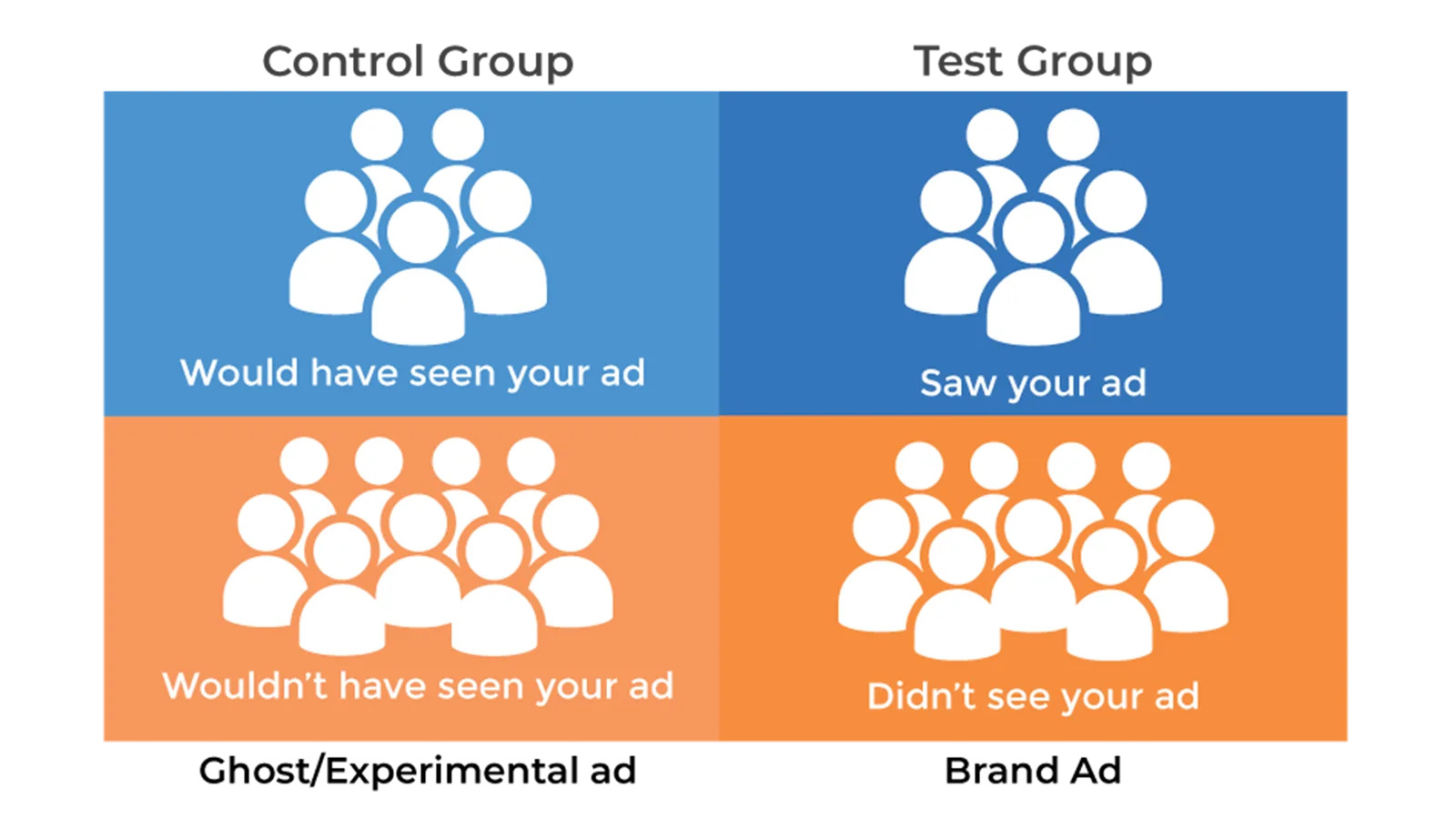
How do you measure uplift in a non-IDFA world?
The above methods are all deterministic in nature i.e., you can not perform them unless you have the user identifier. But in a world that is increasingly privacy-first, how do you measure uplift if you do not have access to IDFA?
That’s when uplift measurement becomes probabilistic through impact measurement methodologies. Here, one must decide how to deal with a test and control group based on available dimensions such as geography, creatives, fingerprinting, persona graphs, and supply-side analytics in order to conduct testing and obtain tangible results.
Any uplift test would require backend data to determine uplift because in the absence of device IDs, no vendor would be able to conduct tests independently on behalf of an advertiser. Hence, close collaboration between the performance channel and advertiser is key to driving research and value through incrementality testing.
Where should you start with incrementality?
One of the factors of resistance to measuring incrementality or uplift is its perceived level of complexity. But with the help of an advertising partner, it is very much possible to conduct regular uplift tests as a standard practice. Here’s how you can do the groundwork:
🔹 Define the key KPIs that measure the success of your campaigns and your business goals.
🔹 From the existing audience set, randomly carve out the test and control group.
🔹 Ensure that you are not running any other campaigns targeting the same set of users to avoid pollution.
🔹 You must have enough budget and reach in comparison to the test audience size so that the test can be statistically significant, i.e., for the results to be considered reproducible.
Keep in mind: If you are running with multiple channels, then clarify the audience for the target group and control group to ensure that the ads are not shown to the control group by any other channel.
Turning the results into guiding insights
Incrementality testing itself is a small piece of a bigger puzzle. As an app marketer who must always justify their investments in different channels, the results from incrementality testing are a moment of truth.
Here’s how you can use the results to guide your next steps:
🔹 Have a clearly outlined primary outcome: Identify the business metrics that define your campaign’s success. The results are entirely dependent on the business goals that you are trying to validate. For eg: A positive conversion rate can give you confidence in your campaigns even though it doesn’t fully translate into a higher ROAS.
🔹 Use your results to scale up appropriate channels and stop cannibalizing traffic: Ensure that your company’s growth targets do not incentivize cannibalization of organic traffic.
🔹 Constantly adapt your hypothesis as results may vary when applied to a larger audience.
Different partners approach these methodologies differently. It is important to work with a trustworthy programmatic DSP partner who guides you through this process and gives you full transparency.
RevX provides incremental uplift measurement enabling advertisers to ascertain the value a channel is adding to their marketing strategy. This way, you are certain that you can intelligently
optimize your campaigns and put money where the impact is.
Reach out to us: https://www.revx.io/